- Home
- The Cult of AI: Perceiving AI to Be More Mature Than It Is
- You Call This Artificial “Intelligence”? AI Meets the Real World
- Turning Lemons into Reflux: When AI Makes Things Worse
- We’re Not Done Yet: After Developing the AI
- Failure to Launch: How People Can React to AI
- AI Registry: The Things We’ll Need That Support AI
- Lessons Learned
AI Registry: The Things We’ll Need That Support AI
AI isn’t just about the data and algorithms. To be successful, we as developers and deployers depend on a whole line of supporting elements. This section addresses some, but not all, of those elements, including the right governing policies, the right people, the right data, and the right equipment.
Explore the Four Fails in This Category:
- Good (Grief!) Governance
- Just Add (Technical) People
- Square Data, Round Problem
- My 8-Track Still Works, So What's the Issue?
Good (Grief!) Governance
We sometimes implement AI without a detailed strategy for how it will be governed, and there aren’t any laws that ensure oversight and accountability. In that vacuum, the technology itself is redefining cultural and societal norms.
Without proper governance, and legal accountability and oversight, the technology becomes the de-facto norm. Therefore, we must recognize that because we control the code, we may unintentionally become de-facto decision makers.
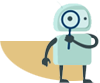
Examples
Police departments can purchase crime prediction products that estimate where crimes will occur or who will be involved. Many of the products are “black boxes,” meaning it is not clear how decisions are made, and many police departments deploy them in the absence of clear or publicly available policies to guide how they should be applied.1 Often a new technology is acquired and used first, while policy and governance for its use are developed later.
Employees of a contractor working for Google paid dark-skinned, homeless people $5 for letting the contractor take a picture of their faces in order to make its training dataset more diverse.2 In addition, these workers may have misled the homeless about the purpose of their participation. Without comprehensive legislation about data collection and privacy infringement, ending such questionable practices becomes the responsibility of the governance policies of each company.
Why is this a fail?
AI has reached a state of maturity where governance is a necessary, yet difficult, element. AI systems continue to be increasingly integrated into daily life, but this occurs without adequate governance, oversight, or accountability. This happens in part because:
1. AI is a probabilistic and dynamic process, meaning AI outcomes will not be fully replicable, consistent, and predictable. Therefore, new governance mechanisms must be developed.
2. Organizations allocate money to buy products, but often do not add funds for creating and testing internal governance policies. Therefore, those policies may not be introduced until the effects of the technology’s use have had an impact on people’s lives.
3. Government and private organizations sometimes keep policies that govern AI use and development hidden from the public in order to protect national security interests or trade secrets.3
4. There are no mature AI laws, standards or norms that apply across multiple domains, and laws within a specific domain are only now emerging. Therefore, standardizing policies or sharing best practices face additional obstacles.
The result is that in the United States there are few clear governance models for industry or government to replicate, and there are limited legal authorities that specify whom to hold accountable when things go wrong.4,5
What happens when things fail?
In response to unclear legal accountabilities, organizations have embraced declarations of ethical principles and frameworks that promote responsible AI development.6 These statements vary in detail and specificity, but almost all declare principles of transparency, non-discrimination, accountability, and safety. These current approaches represent important steps, but evidence shows that they are not enough. They are almost universally voluntary commitments, and few of the declarations include recommendations, specifics, or use cases for how to make the principles actionable and implementable (though in the largest AI companies, these are being developed).7 Finally, researchers have shown that pledges to uphold ethical principles do not guarantee ethical behavior.8
In parallel with private efforts, the US government is beginning to define guidance, but it is still in early stages. In January 2020, the White House published draft principles for guiding federal regulatory and non-regulatory approaches to AI,9 and state governments are also getting more involved in regulation.10 However, often state laws are contradictory or lag the technology. As of January 2020, several cities in California and Massachusetts have banned the use of facial recognition technology by public entities,11 but at the same time other US cities, as well as airports and private entities, are increasing their adoption of the same technology.12,13 Because this field of law is so new there are limited precedents.
Absent precedent, AI applications – or more accurately we, the developers –unintentionally create new norms. The dangers that we must keep in mind are that the AI can undermine traditional figures of authority and reshape the rule of law. Without proper governance, and legal accountability and oversight, the technology becomes the de-facto norm. Therefore, we must recognize that because we control the code, we may unintentionally become de-facto decision makers.14
Just Add (Technical) People
AI skills are in ever-higher demand, but employers erroneously believe that they only need to hire technical people (with backgrounds in computer science, engineering, mathematics, or related fields), even though developing successful and beneficial AI is not purely a technical challenge.
Examples
IBM Watson produced “unsafe and incorrect” cancer treatment recommendations, including “recommendations that conflicted with national treatment guidelines and that physicians did not find useful for treating patients.” Internal IBM documents reveal that training was based on only a few hypothetical cases and a few specialists’ opinions. This finding suggests that including more doctors, hospital administrators, nurses, and patients early in the development process could have led to the use of proper diagnostic guidelines and training data.1
A crash between a US Navy destroyer and an oil tanker resulted from a navigation system interface that was poorly designed, overly complicated, and provided limited feedback.2 Engineers and scientists who study how poor interfaces lead to mishaps can and have helped shape better interface design and safety processes.
In 2015, Google’s automated photo-tagging software mislabeled images of dark-skinned people as “gorillas.” Through 2018, Google’s solution was to remove “gorilla” and the names of other, similar animals from the application’s list of labels.3 Hiring employees and managers trained in diverse disciplines, and not merely technical ones, could have resulted in alternative, more inclusive, outcomes.
Why is this a fail?
The small size of the AI workforce is often cited as the greatest barrier to AI adoption.4 This same problem applies in other fields; for example, healthcare and cybersecurity have similar shortages of skilled technical workers. When responding to the immediate need for AI talent, companies rightly focus on hiring and training data scientists with expertise in AI algorithms, or other specialists in the fields of computer science, engineering, mathematics, and related technical areas. While these employees are absolutely necessary to develop and implement AI at a technical level, just as necessary are specialists from other fields who can balance and contextualize how AI is applied in that domain.
What happens when things fail?
The healthcare and cyber fields are a couple of years ahead of AI when it comes to articulating the skills and abilities necessary for a fully representative workforce. Leaders in both fields recognize that the shortage of technical skills is one challenge, while creating multidisciplinary teams is another. For example, the US government developed a National Initiative for Cybersecurity Education (NICE) framework that “describes the interdisciplinary nature of the cybersecurity workforce [and]… describes cybersecurity work and workers irrespective of where or for whom the work is performed.5 Healthcare organizations have long realized that meeting workforce needs involves more than just hiring doctors and have acted on evidence that interdisciplinary collaboration leads to better patient outcomes.6,7,8
In contrast, the companies and organizations that develop and deploy AI have not yet designed or agreed on similar AI workforce guidelines, though the US government does recognize the importance of interdisciplinary and inclusive teams in several AI strategy publications.9,10 The next step is to move from recognition to implementation.
Square Data, Round Problem
Having data doesn’t mean we have a solution: the right data for the problem is not always easily collectable, or in formats that are ingestible or comparable. What’s more, we may not be able to collect data on all the factors that a given AI application must take into account for adequately understanding the problem space.
Care must be taken to ensure that the obsession for [sic] effectiveness and predictability behind the use of algorithms does not lead to us designing legal rules and categories no longer on the grounds of our ideal of justice, but so that they are more readily ‘codable’
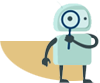
Examples
United Airlines lost $1B in revenue in 2016 from relying on a system that drew on inaccurate and limited data. United had built a software system to forecast demand for passenger seating, but the assumptions behind the data were so flawed and out of date that two-thirds of the system’s outputs were not good enough for accurate projections.1
The Navy, Air Force, and Army all collect different information when they investigate why an aircraft crashes or has a problem, making it difficult for the Department of Defense (DoD) to compare trends or share lessons learned.2
Why is this a fail?
Some AI applications require large amounts of data to be effective. Fortuitously for the AI community, we are experiencing an explosion of data being generated (2.5 quintillion bytes a day, and growing3). But much of this data is not ready for exploitation. The data can be full of errors, leave gaps, or not be standardized, making its practical use challenging (as seen in the United Airlines example). As a result, a surprisingly high number of businesses (79%) are basing critical decisions on data that hasn’t been properly verified.4 On the other hand, valid and useful data can be incompatible across multiple similar applications, preventing an organization from creating a fuller picture (as seen in the DoD example).
What happens when things fail?
The challenge for some of us then, is to understand that more data isn’t a solution to every problem. Aside from concerns over accuracy, completeness, and historical patterns, not all factors can be captured by data. Some of the problem-spaces involved have complex, interrelated factors: for example, one study on community policing found that easy-to-collect data, like the number of crime reports and citations, was used for determining how to combat crime; yet this approach overlooks factors vital to correctly addressing the issues, such as identifying community problems, housing issues, and public health patterns.5
The French Data Protection Authority (the government agency responsible for the protection of personal data) warns against ignoring a complex reality for the sake of results: “care must be taken to ensure that the obsession for [sic] effectiveness and predictability behind the use of algorithms does not lead to us designing legal rules and categories no longer on the grounds of our ideal of justice, but so that they are more readily ‘codable.’”6
My 8-Track Still Works, So What’s the Issue?
Organizations often attempt to deploy AI without considering what hardware, computational resources, and information technology (IT) systems users actually have.
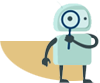
Examples
The Department of Defense still uses 8-inch floppy disks in a system that “coordinates the operational functions of the nation’s nuclear forces.”1 Implementing advanced algorithms would be impossible on this hardware.
95% of ATM transactions still use COBOL, a 58-year-old programming language (numbers as of 2017), which raises concerns about maintaining critical software over the next generation of ATMs.2
Why is this a fail?
The latest processors have amazing computational power, and most AI companies can pay for virtual access to the fastest and most powerful machines in the cloud. Government agencies are often an exception: short-term budget priorities, long and costly acquisition cycles, and security requirements to host their own infrastructure in-house3,4 have pushed the government towards maintaining and sustaining existing IT, rather than modernizing the technology.5 Another exception is established commercial institutions with vital legacy infrastructure (for instance, 92 of the top 100 banks still use mainframe computers), which have such entrenched dependencies that updating IT can have costly and potentially disruptive effects on the business.6
What happens when things fail?
Any group that depends on legacy systems finds it hard to make use of the latest AI offerings, and the technology gap continues to increase over time. While an organization’s current IT may not be as obsolete as the examples here, any older infrastructure has more limited libraries and software packages, and less computational power and memory, than modern systems, and therefore may not meet the requirements of heavy AI processing. So, algorithms developed elsewhere may not be compatible with existing solutions and can’t simply be ported to an older generation of technology.
Add Your Experience! This site should be a community resource and would benefit from your examples and voices. You can write to us by clicking here.